Classifying CIFAR10 images using a ResNet and Regularization techniques in PyTorch
Training an image classifier from scratch to over 90% accuracy in less than 10 minutes
This notebook is an extension to the tutorial Image Classification using CNNs in PyTorch, where we trained a deep convolutional neural network to classify images from the CIFAR10 dataset with around 55% accuracy. In this tutorial, we'll use the following techniques to achieve over 90% accuracy in less than 10 minutes:
- Data normalization
- Data augmentation
- Residual connections
- Batch normalization
- Learning rate annealing
- Weight Decay
- Gradient clipping
Preparing the Data
Let's begin by downloading the dataset and creating PyTorch datasets to load the data, just as we did in the previous tutorial.
import os
import torch
import torchvision
import tarfile
from torchvision.datasets.utils import download_url
# Dowload the dataset
dataset_url = "http://files.fast.ai/data/cifar10.tgz"
download_url(dataset_url, '.')
# Extract from archive
with tarfile.open('./cifar10.tgz', 'r:gz') as tar:
tar.extractall(path='./data')
data_dir = './data/cifar10'
# Look inside the dataset directory
print(os.listdir(data_dir))
classes = os.listdir(data_dir + "/train")
print(classes)
0%| | 0/168168549 [00:00<?, ?it/s]
Downloading http://files.fast.ai/data/cifar10.tgz to ./cifar10.tgz
168173568it [00:01, 100256089.76it/s]
['labels.txt', 'train', 'test']
['airplane', 'deer', 'dog', 'ship', 'frog', 'bird', 'truck', 'horse', 'cat', 'automobile']
There are a few of important changes we'll make while creating the PyTorch datasets:
-
Instead of setting aside a fraction (e.g. 10%) of the data from the training set for validation, we'll simply use the test set as our validation set. This just gives a little more data to train with.
-
We will normalize the image tensors by subtracting the mean and dividing by the standard deviation across each channel. As a result, the mean of the data across each channel is 0, and standard deviation is 1. Normalizing the data prevents the values from any one channel from disproportionately affecting the losses and gradients while training, simply by having a higher or wider range of values that others.
-
We will apply randomly chosen transformations while loading images from the training dataset. Specifically, we will pad each image by 4 pixels, and then take a random crop of size 32 x 32 pixels, and then flip the image horizontally with a 50% probability. Since the transformation will be applied randomly and dynamically each time a particular image is loaded, the model sees slightly different images in each epoch of training, which allows it generalize better.
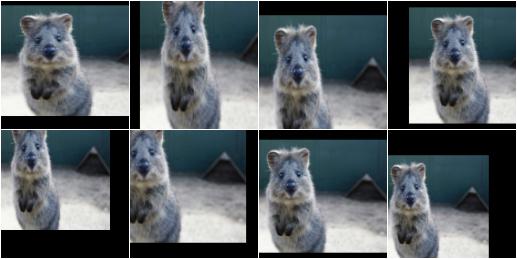